Driver Behavior Profiling for Road Safety
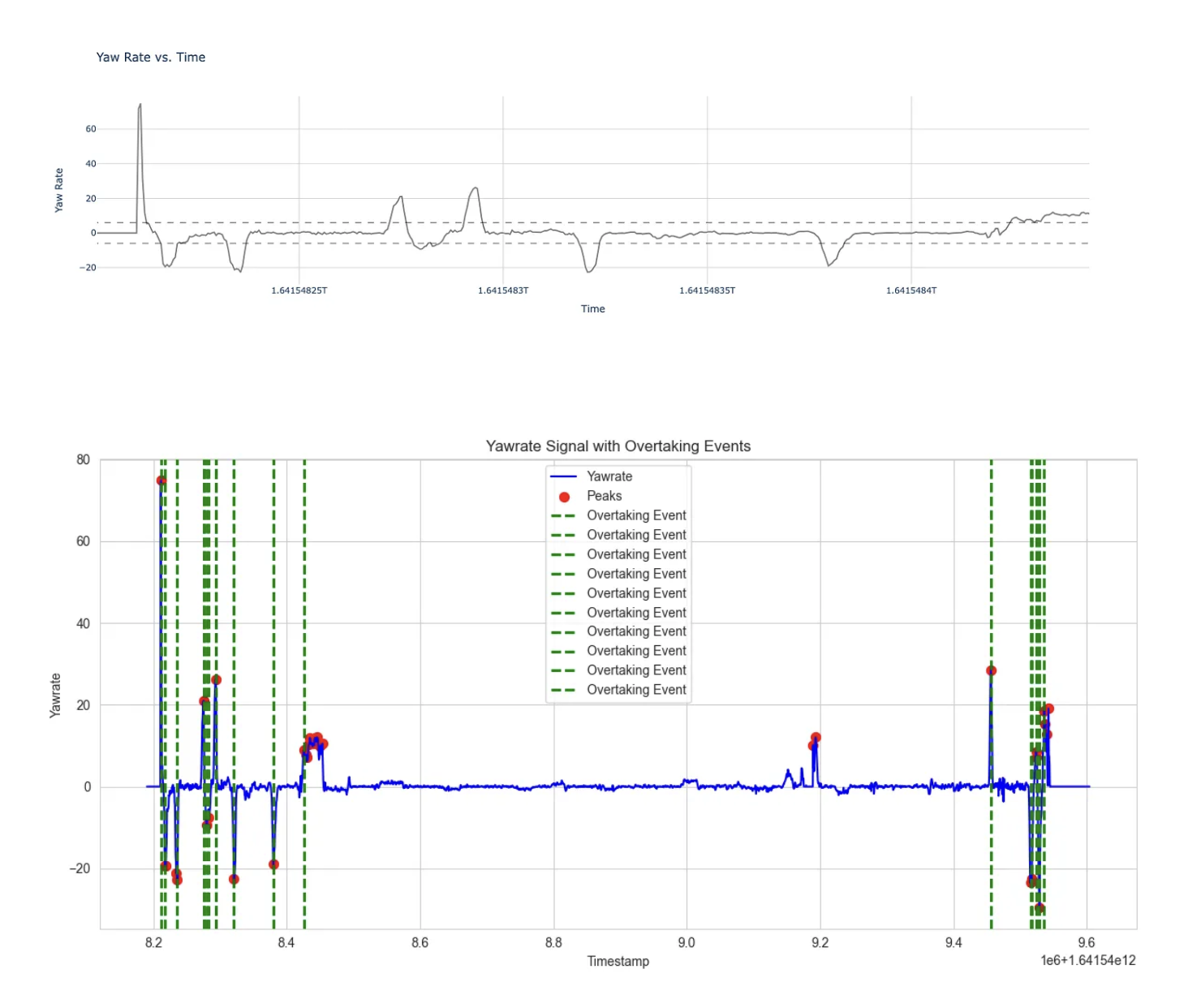
Enhancing Road Safety Through Telematics and Machine Learning
This University of Colorado Boulder's capstone project focused on enhancing road safety by profiling drivers using telematics data. This initiative aimed to understand and improve driver behavior by analyzing factors such as speed, sudden movements, and lane changes. By collecting and examining data from numerous vehicles, the project identified risky driving patterns and suggested areas for improvement. Led by 99P Labs, the effort utilized advanced data analysis techniques to gain deeper insights into how drivers interacted with their vehicles and the roads, ultimately aiming to promote safer driving habits through a better understanding of individual behaviors.
The project began with a comprehensive data collection phase, where telematics data from fleet vehicles were gathered. This data included detailed information on various driving metrics such as acceleration, braking, speed, and route choices. The team cleaned and preprocessed the data to ensure accuracy and reliability for subsequent analysis. This foundational work enabled the team to identify key patterns and trends that could inform their recommendations for improving road safety.
In the analysis phase, the team applied machine learning techniques, specifically KMeans clustering, to categorize drivers into distinct behavior groups. These groups were defined based on their driving styles, such as law-abiding, cautious, and speed enthusiasts. By clustering the drivers, the team could target specific safety interventions more effectively and develop predictive models for identifying potentially risky drivers. Visualizations, including histograms and graphs, were created to illustrate the distribution of driving behaviors and highlight areas of concern.
One of the critical findings was the identification of high-risk driving behaviors, such as frequent sudden movements and excessive speeding. The team used these insights to suggest targeted interventions that could mitigate these risks. For example, drivers exhibiting high-risk behaviors could be provided with personalized feedback and training to improve their driving habits. Additionally, the project explored the potential of using real-time telematics data to provide immediate alerts to drivers when they engage in risky behaviors, further enhancing road safety.
The project concluded with several key recommendations for leveraging telematics data to improve road safety. The team emphasized the importance of continuous monitoring and analysis of driving behaviors to identify emerging risks and trends. They also suggested integrating telematics data with other sources, such as weather and traffic conditions, to develop more comprehensive predictive models. Overall, the project demonstrated the potential of telematics data and machine learning to enhance road safety by providing valuable insights into driver behavior and enabling targeted interventions.
By profiling drivers and analyzing their behaviors through telematics data, this capstone project at the University of Colorado Boulder highlighted the critical role of advanced data analytics in promoting safer driving habits. The insights gained from this study provide a solid foundation for future research and development efforts aimed at improving road safety through innovative use of technology. The project's findings underscore the value of understanding individual driving behaviors and the impact of targeted interventions in reducing road accidents and enhancing overall traffic safety.
Stay Connected
Follow our journey on Medium and LinkedIn.